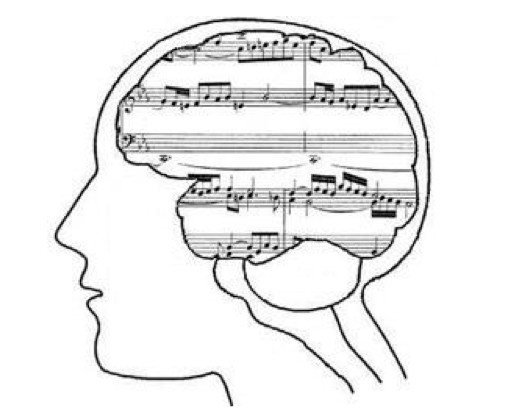
What is the central limit theorem?
Perhaps the most important concept in statistical inference. It gives us two primary insights:
If a variable is normally distributed, the sampling distribution of a test statistic calculated from this variable will also be normally distributed.
If a variable’s distribution is non-normal or unknown, the sampling distribution of its test statistics approach normality as sample size increases.
Why is this important?
Normality assumptions arise frequently in statistics and econometrics. Hypothesis testing relies on a normally distributed sampling distribution to allow us to know the likelihood of a particular test statistic occuring. General linear models require normally distributed residuals. Etc etc etc.
What’s our goal?
Our goal here is to replicate the process of drawing repeated samples from a population with underlying non-normal distribution of our choosing, as a simplified version of this great stack overflow post.
We take t
trials of size n
and store them in a matrix, then calculate the mean of each trial (i.e. row) in a function as such:
sample.means <- function(samps, t, n) {
rowMeans(matrix(samps, nrow = t, ncol = n))
}
On average, our underlying distribution will take on values of one 80% of the time and zero 20% of the time - quite far from normality.
After using set.seed()
with an arbitrary number to ensure reproducibility, we define t
and n
with some “low” values. The rbinom()
function will return random draws of 1 and 0 according to our defined probabilities, which we store in samps
. Finally, we use our sample.means
function above to calculate the average of each trial, for a total of t
sample means.
set.seed(1000)
t <- 150
n <- 50
samps <- rbinom(t*n, size = 1, prob = .8)
samp.means <- sample.means(samps, t, n)
All that remains is to plot histograms of the underlying data samps
and the sample means samp.means
, and voila:
library(ggplot2)
library(gridExtra)
library(plotly)
sample <- qplot(samps, geom = "histogram", bins = 30, main = "")
sample_means <- qplot(samp.means, geom = "histogram", bins = 30, main = "")
p1 <- ggplotly(sample)
p2 <- ggplotly(sample_means)
subplot(p1, p2)
On the left the dummy appears as we expect, with around 20% of observations at 0 and about 80% at 1. The right hand side shows the distribution of sample means, which is a bit too skewed to be considered normal. As per the CLT, let’s ramp up the size of t
& n
and see what happens.
t <- 1500
n <- 500
samps <- rbinom(t*n, size = 1, prob = .8)
samp.means <- sample.means(samps, t, n)
sample <- qplot(samps, geom = "histogram", bins = 30, main = "")
sample_means <- qplot(samp.means, geom = "histogram", bins = 30, main = "")
p1 <- ggplotly(sample)
p2 <- ggplotly(sample_means)
subplot(p1, p2)
With the increased sample, one can see the smooth bell shaped curve emerging on the right-hand side. Hovering over the middle of this distribution will show its center around our defined mean of .8, thanks to the magic of the central limit theorem.
Small Samples?
What can we do if don’t have access to a large sample and our distribution of interest is non-normal or unknown? Bootstrapping is our knight in shining armor here, which will be the subject of a future post.
That’s all for now.
- Louis